Research Summary Report of C06
Integration of Additive Manufacturing in the Construction Process
[18.08.2023]
Mawas, Karam; Doctoral researcher, k.mawas@tu-braunschweig.de
Gerke, Markus; Project leader, m.gerke@tu-braunschweig.de
Maboudi, Mehdi; Associated scientist, m.maboudi@tu-braunschweig.de
all: TU Braunschweig, Institute of Geodesy and Photogrammetry (IGP)
Main goal
To guarantee adherence to a resilient process and faithful realization of the designed model in the printed object, it is essential to implement ongoing and automated data capture and process inspection. Additionally, quality control plays a pivotal role in enabling the seamless integration of components into objects.
Summary
An essential phase within 3D printing is quality assurance. Incorporating automated quality control into the production cycle can significantly augment productivity. With the rapid construction capabilities offered by 3D concrete printing (3DCP), upholding stringent quality standards assumes even greater significance. This highlights the need for the emergence of effective methodologies and concepts to replace labour-intensive manual quality control procedures. Furthermore, it becomes imperative to devise techniques for evaluating the structural attributes of 3D-printed items, ensuring alignment with design specifications and prompt identification of any potential defects, as pointed out by Mechtcherine et al. in 2022.
The composition of the filament holds a major role in the 3D printing process, as its form can be influenced by an array of factors such as the blend of materials, nozzle proximity and dimensions, extrusion pace, pumping pressure, robot velocity, and air pressure. These factors collectively impact the filament’s shape, as discussed by Kloft et al. in 2020. In a study conducted by Slepicka et al. (2022), distinct categories of quality inspections were established, along with their respective applications and significance at various stages of the printing process. The researchers identified filament extraction as an integral aspect of feature-specific examination, concentrating on particular attributes of the printed object.
Figure 1 depicts the projected geometry of the filaments and presents their real condition following the printing of a basic concrete wall. To assess filament quality, a comparison was drawn against manually segmented counterparts. Examination of filament geometry facilitates the utilization of diverse inspection techniques, offering a valuable understanding of the assorted parameters within path planning. Thereby, guaranteeing the attainment of the desired final surface texture.
The current state of research
The printed object matches the digital model but with some discrepancies. The height may not meet expectations, while the sides might need more precise printing, sometimes exceeding the planned width. Flaws appear in the segmented point cloud (fig. 1), with 29 layers instead of 30. Automating labelling speeds up this process, reducing errors and extracting the ‘as-manufactured’ layer surface. This surface extraction updates the digital model, enabling advanced FEM analysis.
Methodology
TLS data capture for Point Cloud (PC); C2M distance application for deviation map considering aliasing; noise reduction with bilateral filter; Canny edge detection and horizontal edge extraction; RANSAC-based line detection in sliding windows. Implementing C2M between the printed specimen and the design model requires alignment. Details on our registration method are in our earlier paper (Mawas et al., 2022). Rasterization needs sufficient point density for sampling, considering aliasing and retaining edge details. Generated image size matches the wall dimensions. Pixel values come from C2M results; missing data may need denoising through interpolation.
The filament’s non-smoothness also along with C2M map, topological islands. By utilizing a bilateral filter reduces noise while preserving edges. Then, Canny edge detection identifies edges followed by morphological horizontal operator to extract only horizontal edges. Isolated edges from Canny are connected using a RANSAC-based for first degree polynomial fitting algorithm.
The RANSAC-based method uses M-estimator sample consensus (MSAC) for straight-line connections, assigning weights to inliers based on residuals for accuracy. The line-fitting process identifies inliers from Canny edges, capturing filament contours beyond basic straight lines.
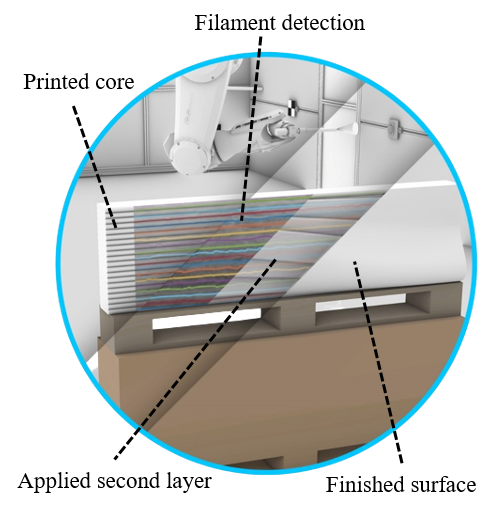
Fig. 1: Extraction of the geometry of filaments and workflow quality inspection from printed core to the finished surface
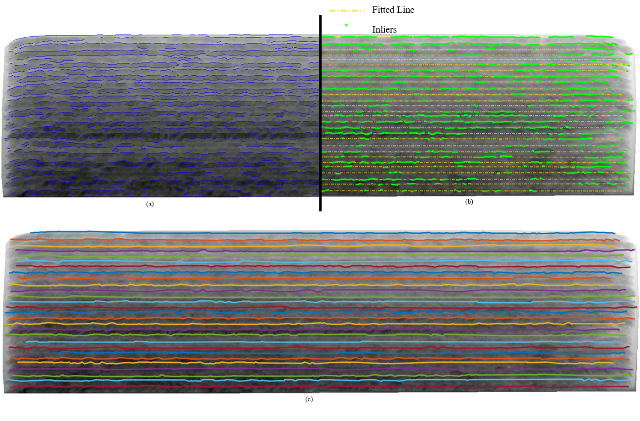
Fig. 2: Extracted edges for every detected filament